Building a comprehensive marketing data warehouse is essential for businesses looking to unlock the power of data-driven marketing strategies. A well-structured data warehouse serves as a central repository for all marketing-related data, enabling businesses to gain valuable insights, make informed decisions, and optimize their marketing campaigns.
In this blog, we will explore the key steps and considerations involved in building a robust marketing data warehouse. From data integration and cleansing to designing a scalable architecture and implementing effective data governance practices, we will provide a comprehensive guide to help businesses create a solid foundation for their marketing analytics initiatives.
What is a Marketing Data Warehouse?
A marketing data warehouse is a centralized repository that stores and organizes marketing-related data from various sources. It is designed to collect, integrate, and consolidate data from multiple channels and systems, such as customer relationship management (CRM) platforms, advertising platforms, email marketing tools, social media platforms, website analytics, and more.
The purpose of a marketing data warehouse is to provide a unified and comprehensive view of marketing data, allowing businesses to analyze and derive insights to drive their marketing strategies.
By aggregating data from diverse sources, a marketing data warehouse enables businesses to gain a holistic understanding of their customers, campaigns, and marketing performance. It allows for the analysis of data across multiple dimensions, such as customer segmentation, campaign effectiveness, channel attribution, and return on investment (ROI).
For example, let's consider an e-commerce company that operates on various digital platforms and marketing channels. They may have data coming in from their website analytics platform, customer purchase history, email marketing campaigns, social media advertising, and more.
A marketing data warehouse would consolidate this data into a single location, making it easier to analyze customer behavior, track the effectiveness of marketing campaigns, measure the impact of different channels, and generate comprehensive reports for decision-making.
Overall, a marketing data warehouse plays a critical role in empowering businesses to make data-driven decisions, optimize marketing efforts, and ultimately achieve better results by leveraging the insights derived from the integrated and organized marketing data.
How to Get Started With Marketing Data Warehousing
Getting started with marketing data warehousing involves several key steps. First, define your objectives and determine the specific marketing data you need to collect and analyze. Next, identify the sources of data and establish data integration processes to bring the data into the warehouse.
Then, design a scalable data architecture and choose the appropriate technology stack. Finally, implement data governance practices to ensure data quality, security, and compliance.
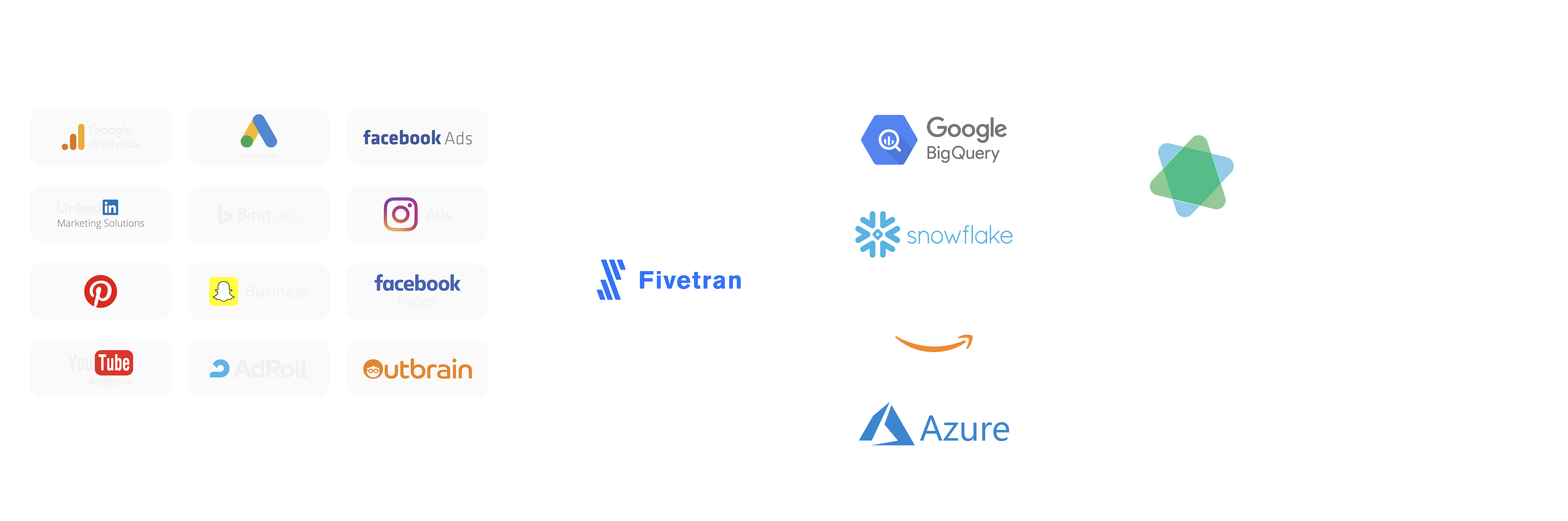
Marketing Data Warehouse Design
Designing a marketing data warehouse involves several crucial steps. First, define your data requirements and identify the relevant sources, such as CRM systems, advertising platforms, and web analytics tools. Next, create a logical data model that organizes your data into dimensions and fact tables.
Choose the right technology stack, including a robust database management system and data integration tools. Implement data governance practices to ensure data quality, security, and compliance.
By following these steps, you can build a well-structured marketing data warehouse design that allows for effective data analysis, insightful marketing insights, and data-driven decision-making to optimize your marketing strategies and drive business success.
Comparison of Data Warehouse With Other Storage Options
When comparing a data warehouse with other storage options, such as data lakes or traditional databases, several key factors come into play. Data warehouses are specifically designed for analytical purposes and offer structured and organized data models that facilitate querying and analysis.
They provide a comprehensive and integrated view of the data, making it easier to derive insights and support decision-making. In contrast, data lakes store vast amounts of raw and unstructured data, allowing for flexible data exploration and experimentation. However, data lakes may require more extensive data preparation and modeling efforts before analysis can take place.
Traditional databases, on the other hand, are primarily designed for transactional processing and may not provide the analytical capabilities and scalability required for robust marketing analytics. Overall, the choice between a data warehouse, data lake, or traditional database depends on the specific needs of the marketing analytics process, including data structure, scalability, and analytical requirements.
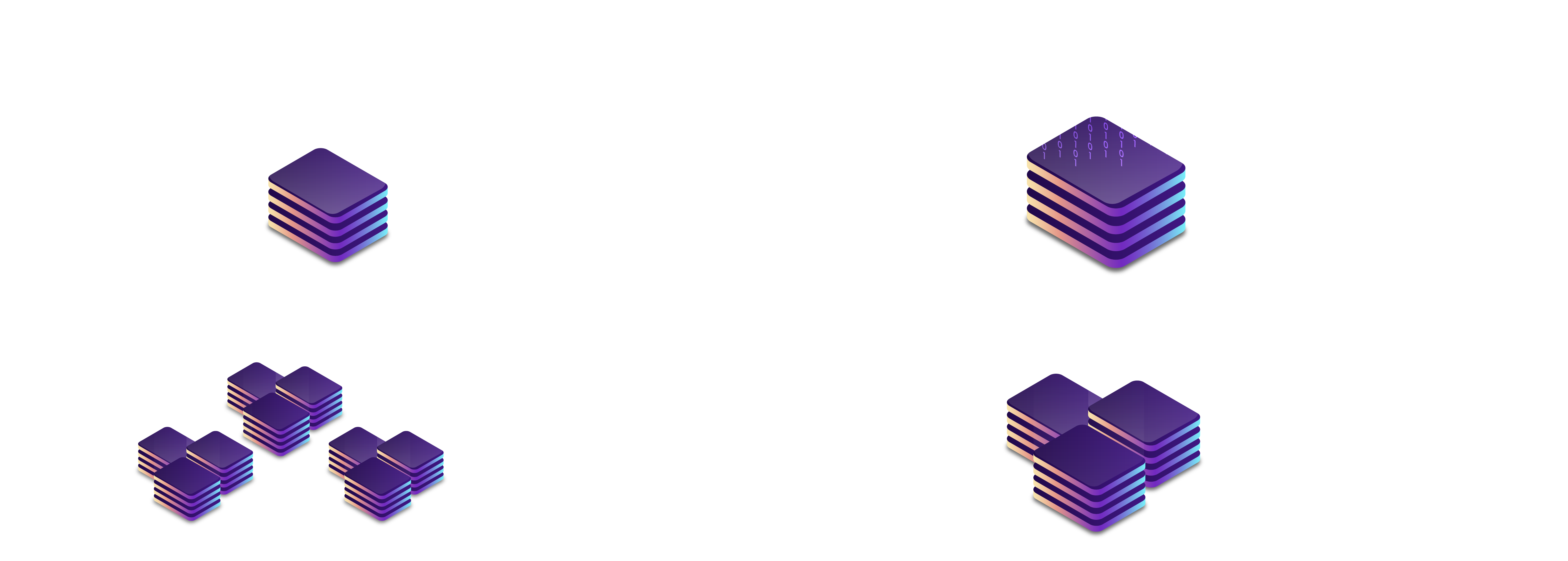
Data Warehouse vs Database vs Data Lake vs Data Marts
Data Warehouse, Database, Data Lake, and Data Marts are different storage options, each serving specific purposes in the realm of data management. A data warehouse is a centralized repository that organizes and integrates data from various sources, providing a comprehensive view for analytical purposes.
A database, typically a relational database, stores structured data and is designed for efficient transactional processing. A data lake, on the other hand, is a storage system that stores raw and unstructured data, enabling flexible exploration and analysis.
Data marts are subsets of data warehouses that focus on specific business functions or departments, providing localized and tailored data for targeted analysis. The choice between these options depends on the nature of data, analytical needs, and the specific use cases of the organization.
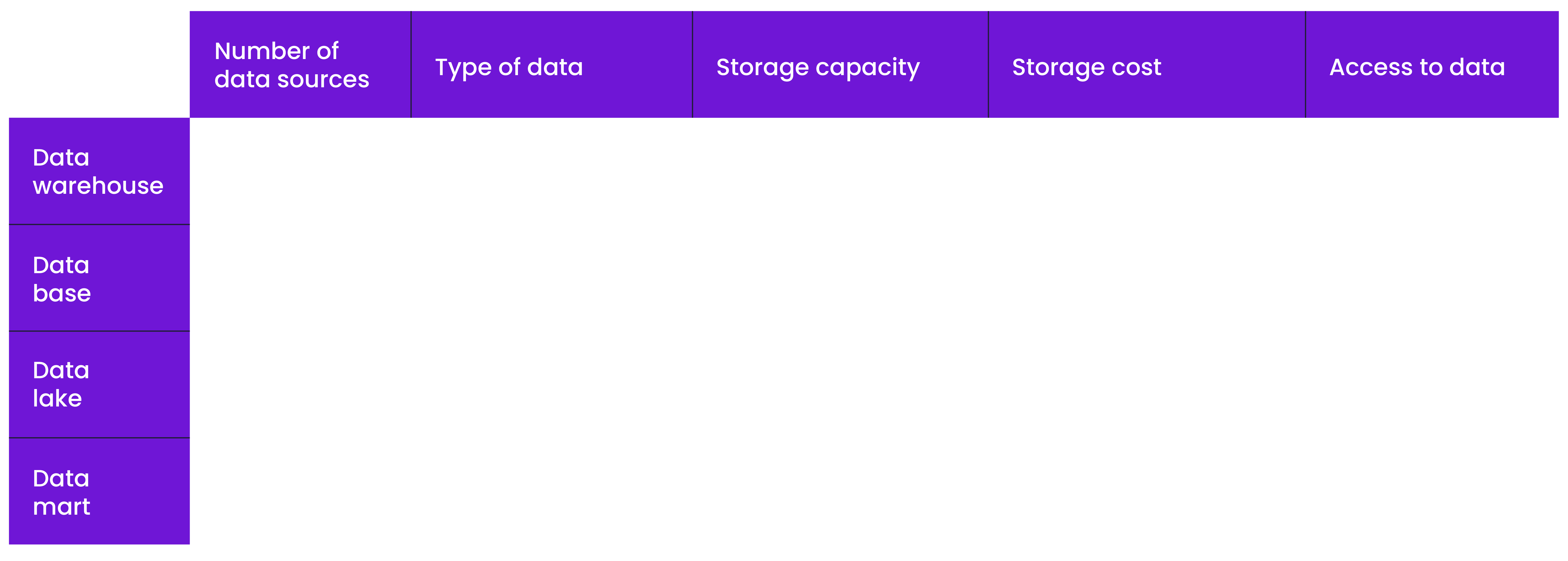
Benefits of Data Warehouse In Marketing
Centralized Data Storage
A data warehouse serves as a centralized repository, consolidating data from various sources such as CRM systems, web analytics tools, and advertising platforms.
This centralized storage enables marketers to have a comprehensive and unified view of their marketing data, making it easier to access, manage, and analyze.
Improved Data Quality
Data warehouses include data cleansing and transformation processes that enhance the quality of the data.
By standardizing and validating the data, marketers can rely on accurate and consistent information for their marketing analytics and decision-making.
Enhanced Customer Segmentation
Data warehouses enable advanced customer segmentation by combining various data points such as demographics, purchase history, browsing behavior, and engagement metrics.
Marketers can create more targeted and personalized marketing campaigns by identifying distinct customer segments based on their characteristics and behaviors.
Deeper Customer Insights
With a data warehouse, marketers can gain deeper insights into customer behavior, preferences, and buying patterns.
By analyzing the integrated data, marketers can understand customer journeys, identify touchpoints that influence purchasing decisions, and tailor marketing strategies accordingly.
Predictive analytics and Forecasting
Data warehouses provide a solid foundation for predictive analytics and forecasting models. Marketers can leverage historical data, combined with advanced analytics techniques, to predict future trends, customer behavior, and campaign performance, aiding in strategic decision-making.
Data Modeling and Setting Up Data Transfers
Data warehouses require effective data modeling to structure the data for analytical purposes. Marketers can design data models that align with their marketing goals and establish automated data transfers from different sources to the warehouse, ensuring regular updates of the data.
Analysis and Reporting
Data warehouses offer powerful analytical capabilities, allowing marketers to perform in-depth analysis on their marketing data.
Marketers can generate comprehensive reports, create custom dashboards, and visualize key metrics, enabling them to monitor campaign performance, measure ROI, and make data-driven decisions.
How can Arena Help in creating a Marketing Data Warehouse
Arena can be instrumental in creating a marketing data warehouse by providing the necessary tools and features to gather, store, and analyze marketing data in a centralized and structured manner. Here's how Arena can assist in building a marketing data warehouse:
- Data Integration: Arena offers data integration capabilities that allow you to connect and consolidate data from various marketing channels and platforms. It can collect data from sources like social media platforms, CRM systems, email marketing tools, advertising platforms, and more, ensuring all relevant marketing data is captured.
- Data Storage and Management: Arena provides a secure and scalable environment to store and manage your marketing data. It offers a robust infrastructure and storage capabilities to handle large volumes of data effectively. With its data governance features, you can maintain data quality, security, and compliance standards.
- Data Transformation and Cleansing: Arena allows you to transform and cleanse your marketing data to ensure its accuracy and consistency. It provides data manipulation features and tools to standardize formats, clean up discrepancies, and handle data inconsistencies, making the data warehouse more reliable and actionable.
- Data Analysis and Reporting: Arena equips you with powerful analytics and reporting features to derive insights from your marketing data. It offers advanced querying and visualization capabilities to explore trends, track campaign performance, identify patterns, and measure key marketing metrics. With interactive dashboards and customizable reports, you can communicate the insights effectively to stakeholders.
By utilizing Arena for your marketing data warehouse, you can centralize and organize your marketing data, enabling a holistic view of your marketing efforts. This consolidated data empowers you to make data-driven decisions, optimize marketing strategies, and uncover valuable insights to drive business growth. Arena's user-friendly interface and robust functionalities make it a valuable tool for creating and maintaining a marketing data warehouse that fuels your marketing analytics and informs your marketing strategies effectively.
Conclusion
Building a comprehensive marketing data warehouse is a crucial step towards harnessing the power of data-driven marketing. By following the key steps and considerations outlined in this guide, businesses can establish a robust foundation for their marketing analytics initiatives. From defining data requirements and integrating diverse data sources to designing a scalable architecture and implementing effective data governance practices, each aspect contributes to the successful development of a comprehensive marketing data warehouse.
By investing time and resources in building a data warehouse, businesses can unlock valuable insights, optimize marketing campaigns, and make informed decisions that drive growth and success.
The centralized storage, improved data quality, enhanced customer segmentation, deeper customer insights, predictive analytics capabilities, and efficient analysis and reporting offered by a data warehouse empower marketers to maximize the impact of their marketing strategies.
Take the first step towards building a comprehensive marketing data warehouse and unlock the full potential of your marketing efforts. Start by defining your objectives, understanding your data requirements, and implementing the necessary steps outlined in this guide.
With a well-designed and fully-functional data warehouse, you can revolutionize your marketing approach and stay ahead in today's data-driven business landscape.
Begin your journey towards building a comprehensive marketing data warehouse today and revolutionize your marketing strategies. Contact us to learn more about how our expertise and solutions can help you achieve your data-driven marketing goals.
FAQs on Marketing Data Warehouse
What are the 5 key components of the data warehouse?
The five key components of a data warehouse are:
- Data Sources: These are the systems, databases, and applications that provide the raw data to be integrated into the data warehouse. Data can be sourced from various internal and external systems, such as CRM platforms, transactional databases, marketing automation tools, and external data providers.
- Data Integration: Data integration involves the processes and techniques used to extract, transform, and load (ETL) the data from different sources into the data warehouse. This includes data cleansing, data transformation, and data consolidation to ensure consistency and quality.
- Data Storage: The data storage component encompasses the physical infrastructure and technologies used to store the integrated data. This typically involves a database management system (DBMS) optimized for analytical processing, such as a relational database or a columnar database, that can efficiently store and retrieve large volumes of data.
- Data Modeling: Data modeling involves designing the structure and organization of the data within the data warehouse. This includes creating dimensional models, such as star schemas or snowflake schemas, to represent the relationships between data entities and facilitate efficient querying and analysis.
- Data Access and Analysis: The data access and analysis component provides tools and mechanisms for users to query, analyze, and visualize the data within the data warehouse. This can include business intelligence (BI) tools, reporting tools, ad hoc query tools, and data visualization tools that enable users to explore and derive insights from the data.
These key components work together to create a comprehensive data warehouse environment that supports data integration, storage, modeling, and analysis, enabling businesses to leverage their data for strategic decision-making and analytics.
What are the 4 stages of the data warehouse?
The four stages of the data warehouse are:
- Requirement Gathering: In this stage, businesses identify and gather the requirements for their data warehouse. This involves understanding the organization's goals, objectives, and data needs. It also includes identifying the key data sources, determining the scope of the data warehouse, and defining the data requirements for analysis and reporting.
- Data Modeling and Design: This stage involves designing the structure and organization of the data within the data warehouse. Data modeling techniques, such as dimensional modeling, are used to create a logical representation of the data and define the relationships between different entities. This stage also includes designing the physical storage infrastructure, selecting the appropriate database management system, and establishing data integration processes.
- Implementation and ETL: In this stage, the data warehouse is implemented based on the design specifications. This involves setting up the physical infrastructure, configuring the database management system, and building the ETL (Extract, Transform, Load) processes. Data extraction mechanisms are established to extract data from various sources, transform it into the desired format, and load it into the data warehouse.
- Testing and Deployment: Once the data warehouse is implemented, it goes through rigorous testing to ensure its functionality, accuracy, and performance. This stage involves testing the ETL processes, validating the data quality, and conducting performance testing. Once the data warehouse passes the testing phase, it is deployed and made accessible to users for querying, analysis, and reporting.
These four stages form a systematic approach to building a data warehouse, starting from gathering requirements to designing the structure, implementing the infrastructure, and finally testing and deploying the data warehouse for productive use.
Which data comes first to build a data warehouse?
To build a data warehouse, the data that comes first is typically the data requirements and understanding the business needs. It is essential to determine what data is needed for analysis and reporting purposes, and how it aligns with the organization's goals and objectives. This involves gathering information from stakeholders, understanding their reporting and analysis requirements, and identifying the key data sources that will provide the necessary data.
Once the data requirements are defined, the next step is to gather and integrate the relevant data from various sources. This can include internal systems such as CRM platforms, transactional databases, and operational systems, as well as external data sources such as third-party providers or data feeds. The data integration process involves extracting the data from these sources, transforming it into a standardized format, and loading it into the data warehouse.
In summary, understanding the data requirements and aligning them with the business needs is the first step in building a data warehouse. This is followed by the gathering and integration of the relevant data from multiple sources to create a comprehensive and unified view of the data within the data warehouse.
What is the 9-step method to design a data warehouse?
The 9-step method to design a data warehouse typically follows these stages:
- Identify Business Requirements: Understand the business objectives, reporting and analysis needs, and key performance indicators (KPIs) that the data warehouse should support. This involves engaging with stakeholders and conducting thorough business analysis.
- Define Data Requirements: Determine the specific data elements, attributes, and metrics required to fulfill the business requirements. Identify the relevant data sources and understand the relationships between data entities.
- Create a Conceptual Data Model: Develop a high-level representation of the data warehouse structure, focusing on entities, relationships, and business concepts. This provides a conceptual framework for organizing and integrating the data.
- Design the Logical Data Model: Create a detailed logical data model that translates the conceptual model into a relational or dimensional model. Define the tables, attributes, primary and foreign keys, and establish relationships between tables.
- Select Data Warehouse Architecture: Choose an appropriate data warehouse architecture, such as a centralized, decentralized, or hybrid model, based on factors like data volume, complexity, and performance requirements.
- Choose the Technology Stack: Select the necessary hardware, database management system (DBMS), ETL (Extract, Transform, Load) tools, and reporting and analysis tools that align with the data warehouse design and requirements.
- Develop ETL Processes: Design and implement the ETL processes to extract data from source systems, transform it into the desired format, and load it into the data warehouse. This involves mapping data fields, performing data cleansing, and applying necessary transformations.
- Build the Data Warehouse Schema: Create the physical database schema based on the logical data model. Define the tables, columns, indexes, and partitions needed to store the data efficiently and optimize query performance.
- Implement Reporting and Analysis: Develop reporting and analysis components that enable users to query, analyze, and visualize the data within the data warehouse. This includes creating dashboards, reports, and ad hoc query tools that cater to the specific reporting and analysis requirements of the business users.
By following these steps, organizations can design a well-structured and effective data warehouse that supports their business objectives and empowers data-driven decision-making.