Machine learning has revolutionized the field of predictive analytics, empowering organizations to uncover valuable insights from vast amounts of data. As the volume and complexity of data continue to grow exponentially, traditional statistical methods are often insufficient to extract meaningful patterns and make accurate predictions.
This is where machine learning steps in, leveraging sophisticated algorithms and computational power to unlock the potential hidden within data and provide valuable predictive capabilities. Here are some interesting facts about machine learning predictive analytics:
- The global machine learning market is projected to reach $117.19 billion by 2027, indicating the widespread adoption and recognition of its value across various industries.
- Predictive analytics powered by machine learning has been successfully applied in numerous domains, including finance, healthcare, marketing, and cybersecurity, leading to improved efficiency, cost savings, and enhanced customer experiences.
- Machine learning models can continuously learn and adapt from new data, allowing for dynamic and accurate predictions, even in rapidly changing environments.
In this article, we’ll learn everything about machine learning predictive analytics, how it works and its benefits.
What is Predictive Analytics?
Predictive analytics is a branch of data analytics that utilizes historical and real-time data to make predictions about future events or outcomes. It involves applying statistical algorithms, machine learning techniques, and data mining to analyze patterns, identify trends, and forecast future probabilities.
By examining past data, predictive analytics aims to provide insights into what is likely to happen in the future, enabling organizations to make informed decisions and take proactive measures.
Predictive analytics is used across various industries and domains. It helps businesses optimize operations, enhance customer experiences, mitigate risks, and drive strategic planning. From predicting customer behavior and preferences to forecasting market demand, predicting equipment failures, or identifying fraudulent activities, predictive analytics empowers organizations to leverage data-driven insights and gain a competitive advantage in today's dynamic and fast-paced business landscape.
Importance of Predictive Analytics
Predictive analytics is of paramount importance in modern business environments. It enables organizations to anticipate future trends, patterns, and behaviors by leveraging historical and real-time data. By making data-driven decisions, businesses can minimize risks, optimize resource allocation, and stay ahead of the competition.
Additionally, predictive analytics enhances operational efficiency by streamlining processes and identifying areas for improvement. It also enables organizations to provide personalized customer experiences, leading to increased satisfaction and loyalty.
Moreover, predictive analytics plays a crucial role in risk mitigation, fraud detection, and cybersecurity, ensuring the protection of assets and operational continuity. Overall, predictive analytics empowers businesses to leverage data-driven insights, make informed decisions, and gain a competitive advantage in today's fast-paced and data-rich business landscape.
Significance of Machine Learning in Predictive Analytics
Machine learning plays a crucial role in enhancing the effectiveness of predictive analytics. It brings unique capabilities to the table, such as uncovering complex patterns within large and intricate datasets.
Unlike traditional statistical methods, machine learning algorithms can identify subtle relationships and interactions, leading to more accurate predictions and deeper insights. Additionally, machine learning enables automated model training and adaptation, eliminating the need for manual intervention and ensuring that predictive models remain up to date and effective in dynamic environments.
The ability of machine learning algorithms to handle big data and process it at scale is invaluable, allowing for real-time predictions and timely decision-making. Moreover, machine learning algorithms leverage advanced statistical techniques and optimization algorithms to improve predictive accuracy, capturing more nuanced patterns and enhancing the reliability of predictions.
Overall, machine learning significantly enhances the significance of predictive analytics by unlocking its potential to extract valuable insights and make informed decisions based on data-driven predictions.
Advantages of Utilizing Machine Learning in Predictive Analytics
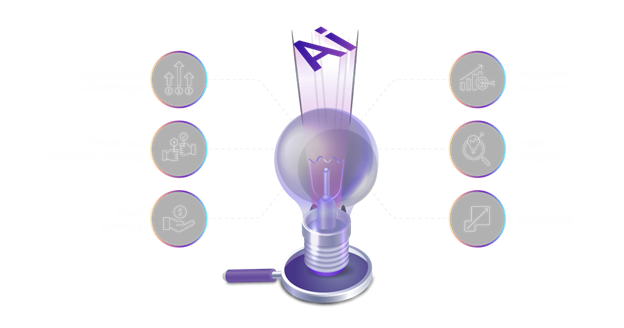
Improved Accuracy
Machine learning algorithms significantly improve the accuracy of predictive analytics by leveraging advanced statistical techniques and adaptive learning. These algorithms can detect complex patterns and relationships within data that may not be apparent to human analysts, resulting in more precise and reliable predictions.
Faster Insights
Machine learning enables faster insights by automating the process of model training and adaptation. These algorithms can quickly process and analyze large volumes of data, providing real-time predictions and insights. This speed allows organizations to make timely decisions and respond swiftly to changes in the market or operational conditions.
Scalability
Machine learning algorithms offer scalability, allowing organizations to handle large and diverse datasets without compromising performance. They can efficiently process and analyze massive amounts of data, making it possible to scale predictive analytics initiatives as data volumes continue to grow.
This scalability ensures that predictive models can handle increased complexity and maintain their effectiveness as the organization expands.
Competitive Advantage
Utilizing machine learning in predictive analytics provides organizations with a competitive advantage. By leveraging advanced algorithms and data-driven insights, businesses can gain deeper understanding and foresight into customer behavior, market trends, and emerging opportunities.
This enables organizations to make proactive decisions, identify new business opportunities, and outperform competitors in the market.
Enhanced Decision-Making
Machine learning empowers organizations to make enhanced and data-driven decisions. By leveraging predictive analytics, businesses can access accurate and timely insights that support informed decision-making.
These insights enable organizations to optimize resource allocation, identify risks, and seize opportunities, leading to better overall decision-making and strategic planning.
Cost Savings
Machine learning in predictive analytics can lead to significant cost savings for organizations. By accurately predicting demand, optimizing inventory management, or identifying operational inefficiencies, businesses can reduce costs associated with overstocking, underutilized resources, or unplanned downtime.
Moreover, machine learning enables automated processes, reducing the need for manual intervention and saving time and labor costs.
Machine Learning Techniques Used in Predictive Analytics
In predictive analytics, several machine learning techniques are commonly used to analyze historical data and make predictions. One widely used technique is linear regression, which establishes a linear relationship between the input features and the target variable to predict numeric values.
Another popular technique is decision trees, which use a tree-like structure to make predictions by splitting the data based on different features. Additionally, ensemble methods like random forests and gradient boosting are frequently employed to combine the predictions of multiple models to improve accuracy.
These techniques leverage large amounts of data to uncover patterns and make informed predictions about future outcomes.
Applications of Machine Learning Predictive Analytics
Predicting Customer Behaviour
Machine learning predictive analytics can be used to analyze customer data and predict their behavior, such as their likelihood to churn, purchase a product, or engage with marketing campaigns.
By leveraging historical data and patterns, businesses can personalize their marketing efforts, improve customer retention, and optimize customer experiences.
Predicting Business Trends
Machine learning predictive analytics can analyze vast amounts of data from various sources to identify emerging trends and patterns in the business landscape. This can help businesses make informed decisions, such as predicting market demand, identifying new opportunities, optimizing inventory management, and staying ahead of competitors.
By leveraging predictive analytics, businesses can gain a competitive edge and adapt to changing market dynamics effectively.
Predicting Medical Outcomes
Machine learning predictive analytics can analyze patient data, medical records, and clinical research to predict medical outcomes such as disease progression, treatment effectiveness, and patient risks. This can aid healthcare professionals in making more accurate diagnoses, developing personalized treatment plans, and improving patient care.
Predictive analytics can also help identify potential outbreaks, optimize resource allocation, and support medical research for improved healthcare decision-making.
Use Cases of Machine Learning Predictive Analytics
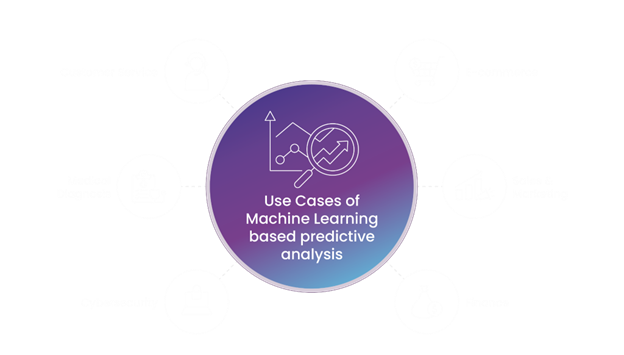
E-Commerce
Machine learning predictive analytics can be applied in e-commerce to personalize product recommendations, predict customer preferences, and optimize pricing strategies. By analyzing customer behavior, purchase history, and product attributes, businesses can offer tailored recommendations, increase conversion rates, and enhance the overall shopping experience.
Customer Service
Machine learning predictive analytics can assist customer service teams in predicting customer needs, sentiment analysis, and optimizing support workflows. By analyzing customer interactions, feedback, and historical data, businesses can anticipate customer issues, provide proactive support, and streamline the resolution process, leading to improved customer satisfaction and retention.
Medical Diagnosis
Machine learning predictive analytics can aid in medical diagnosis by analyzing patient data, symptoms, and medical history to predict potential diseases, treatment outcomes, and personalized therapies.
This technology can support healthcare professionals in making accurate diagnoses, reducing misdiagnosis rates, and enabling early detection and intervention, ultimately improving patient outcomes and reducing healthcare costs.
Sales and Marketing
Machine learning predictive analytics can enhance sales and marketing strategies by analyzing customer data, market trends, and historical sales patterns to predict customer behavior, identify target segments, and optimize marketing campaigns.
This enables businesses to tailor their messaging, allocate resources efficiently, and generate higher conversion rates, leading to improved sales performance and customer engagement.
Financial Services
Machine learning predictive analytics can be used in the financial industry for fraud detection, credit scoring, and risk assessment. By analyzing vast amounts of financial data, transaction patterns, and customer behavior, machine learning algorithms can identify suspicious activities, predict creditworthiness, and assess potential risks, helping financial institutions make informed decisions, minimize fraud, and improve overall operational efficiency.
Cybersecurity
Machine learning predictive analytics plays a crucial role in cybersecurity by analyzing network traffic, user behavior, and system logs to detect and predict potential security threats. By leveraging machine learning algorithms, businesses can identify patterns of malicious activities, predict emerging threats, and take proactive measures to prevent cyber-attacks, ensuring the protection of sensitive data and maintaining a secure digital environment.
Conclusion
Machine learning predictive analytics is a powerful tool that combines the capabilities of machine learning and data analytics to make accurate predictions and drive informed decision-making. By analyzing historical data, identifying patterns, and building predictive models, businesses can gain valuable insights into customer behavior, market trends, and future outcomes.
The benefits of machine learning predictive analytics include improved forecasting, enhanced customer targeting, optimized resource allocation, and increased operational efficiency. To leverage the power of machine learning predictive analytics, we recommend using Arena Calibrate, our cutting-edge predictive analytics tool.
Arena Calibrate offers advanced algorithms, intuitive interfaces, and potent data visualization features to help businesses uncover hidden insights, make data-driven predictions, and take strategic actions. With Arena Calibrate, you can unlock the full potential of your data and stay ahead in today's competitive landscape. Take the next step in your data-driven journey and try Arena Calibrate today.
FAQs on Machine Learning Predictive Analytics
Why is machine learning best for prediction?
Machine learning is best for prediction because it can handle large and complex datasets, discover intricate patterns, and adapt to changing conditions. It leverages advanced algorithms that learn from data and improve over time, enabling accurate predictions based on historical patterns and trends.
Which type of machine learning is used for prediction?
Supervised learning is commonly used for prediction in machine learning. In supervised learning, the model learns from labeled examples to make predictions on new, unseen data. Regression and classification are two common types of supervised learning algorithms used for prediction tasks.
What is good prediction accuracy using machine learning?
The definition of good prediction accuracy varies based on the specific problem and domain. Generally, higher prediction accuracy is desirable. However, what is considered "good" depends on factors such as the complexity of the problem, the quality of the data, and the specific requirements of the application. Evaluation metrics like accuracy, precision, recall, and F1 score are used to measure prediction accuracy.
What is prediction risk in machine learning?
Prediction risk in machine learning refers to the possibility of incorrect or inaccurate predictions. It represents the uncertainty associated with making predictions on new, unseen data. Prediction risk can arise from various factors, including limitations in the training data, model complexity, overfitting, or inherent variability in the target variable. Minimizing prediction risk is a key goal in machine learning to ensure the reliability and usefulness of predictions.