In the rapidly evolving field of data analysis and business intelligence, selecting the right data mining tools and software is crucial for organizations seeking to derive valuable insights from their data. As we enter the year 2023, there are several exceptional data mining software and software available that offer advanced functionalities and enhanced capabilities.
These tools are designed to help businesses efficiently extract patterns, uncover hidden relationships, and make data-driven decisions. With their user-friendly interfaces and powerful algorithms, these tools enable users to process large volumes of data and gain valuable business intelligence.
By leveraging data mining tools, businesses can unlock the full potential of their data, leading to improved efficiency, increased revenue, and better overall decision-making. Hence, in this blog, we’ll cover the top 9 data mining software you should consider using in 2023.
What is Data Mining?
Data mining is the process of extracting meaningful and actionable information from large volumes of data. It involves discovering patterns, relationships, and insights that can help organizations make informed decisions and gain a competitive advantage.
The stages of data mining typically include data collection, data preprocessing, model building, evaluation, and interpretation of results.
What are Data Mining Tools?
Data mining tools are software applications or platforms that facilitate the process of data mining. These tools provide a range of functionalities and algorithms designed to extract meaningful patterns, relationships, and insights from large datasets.
They help users explore, analyze, and interpret data in a more efficient and effective manner. Data mining software often offer features such as data visualization, statistical analysis, predictive modeling, clustering, and classification.
They enable users to preprocess and clean the data, apply various algorithms to uncover patterns, and evaluate the results. These tools may also provide automation and scalability, allowing users to handle large volumes of data and perform complex analyses with ease.
By utilizing data mining software, organizations can accelerate the process of extracting insights from their data, improve decision-making, and gain a deeper understanding of their business operations and customer behavior.
These tools are invaluable in industries such as finance, healthcare, marketing, retail, and many others, where data-driven insights play a crucial role in driving success and achieving competitive advantages.
Essential Data Mining Tools to Familiarize Yourself Within 2023
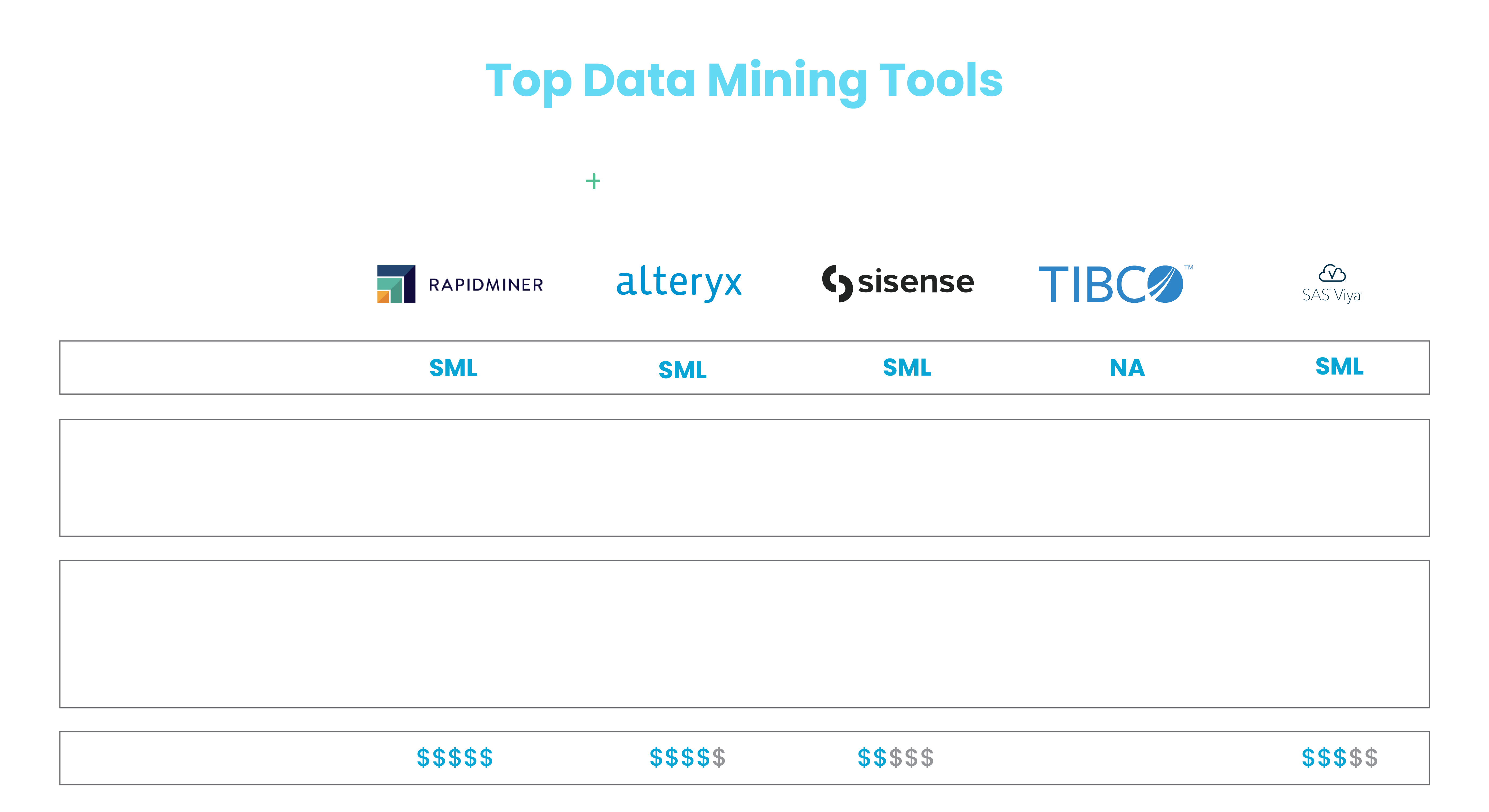
Arena Calibrate
Arena Calibrate is a powerful data mining tool designed to help businesses extract valuable insights and patterns from large and complex datasets.
With its advanced algorithms and user-friendly interface, Arena Calibrate empowers users to uncover hidden relationships, make data-driven decisions, and optimize business strategies.
Key Features
- Data Exploration: Dive deep into your datasets and explore the underlying patterns, trends, and correlations.
- Predictive Analytics: Utilize machine learning algorithms to predict future outcomes and trends based on historical data.
- Clustering and Classification: Group similar data points together and classify them into distinct categories for better understanding and decision-making.
- Visualization: Present your findings through intuitive charts, graphs, and interactive visualizations to facilitate understanding and communication.
- Data Preprocessing: Clean and transform raw data to ensure accuracy and reliability in subsequent analysis.
Use Cases
- Market Research: Identify market trends, customer preferences, and competitor insights to drive strategic decision-making.
- Customer Segmentation: Divide customers into distinct segments based on behavior, demographics, or preferences for targeted marketing campaigns.
- Demand Forecasting: Predict future demand patterns to optimize inventory management, production planning, and supply chain operations.
- Sentiment Analysis: Analyze customer feedback and sentiment to gauge brand reputation, improve customer satisfaction, and guide product development.
User Interface
The Arena Calibrate user interface offers a clean and intuitive design, allowing users to navigate effortlessly through the various features and functionalities. The interface provides easy access to data exploration tools, visualization options, and advanced analytics techniques.
With its user-friendly layout and interactive elements, users can seamlessly interact with their data, explore insights, and generate meaningful visualizations.
Pricing
Arena offers 3 comprehensive price plans, which ensures businesses of all sizes can make the most of the tool. You can start exploring the tools benefits using Arena Lite, which is a free version.
For more advanced features, you can either choose the Arena Business Pro or Arena Business Agency plans, which are customized based on your requirements.
Pros
- Powerful data mining capabilities to extract valuable insights from complex datasets.
- User-friendly interface that facilitates easy exploration, analysis, and visualization of data.
- Advanced algorithms and machine learning techniques for predictive analytics.
- Integration options with various data sources and automation capabilities for streamlined workflows.
- Use cases across multiple industries, including market research, customer segmentation, and fraud detection.
Cons
- Advanced features may require a learning curve for users new to data mining.
- Limited customization options in terms of UI design and functionality.
- Reliance on the quality and availability of data sources for accurate analysis.
RapidMiner Studio
RapidMiner Studio is a popular data mining tool that provides a comprehensive environment for data preparation, modeling, evaluation, and deployment. It offers a visual interface and a wide range of advanced analytics and machine learning algorithms, making it suitable for both novice and experienced users.
Key Features
- Drag-and-drop interface for easy workflow creation.
- Extensive collection of pre-built operators for data preprocessing, modeling, and evaluation.
- Integration with popular programming languages such as R and Python.
- Automated model optimization and feature selection.
- Advanced data visualization capabilities.
- Collaboration and sharing functionalities for team projects.
Use Cases
- Predictive analytics and forecasting.
- Customer segmentation and targeted marketing.
- Fraud detection and anomaly detection.
- Text mining and sentiment analysis.
- Market basket analysis and recommendation systems.
User Interface
RapidMiner Studio offers a user-friendly visual interface that allows users to construct data workflows through a drag-and-drop approach. This interface simplifies the process of creating complex data mining processes, making it accessible to users with varying levels of technical expertise.
Pricing
RapidMiner Studio offers various pricing options, including both free and paid versions. The pricing structure is flexible and based on the specific needs and requirements of the user or organization. Detailed pricing information can be obtained directly from the RapidMiner website.
Pros and Cons
Pros:
- Intuitive and visually appealing interface.
- Extensive library of operators and algorithms.
- Seamless integration with external programming languages.
- Robust data preprocessing capabilities.
- Active community and user support.
Cons:
- Steep learning curve for beginners.
- Limited support for big data processing.
- Some advanced features may require programming knowledge.
- The free version has limitations on the number of data rows and computational resources.
KNIME
KNIME (Konstanz Information Miner) is an open-source data analytics platform that allows users to manipulate, analyze, and model data through a visual workflow-based approach. It provides a wide range of tools and functionalities for data preprocessing, machine learning, data mining, and integration with other data sources.
Key Features
- Drag-and-drop interface for constructing data workflows.
- Extensive collection of data processing and analysis nodes.
- Integration with various programming languages and data sources.
- Workflow automation and batch processing capabilities.
- Collaboration and sharing functionalities.
- Scalability for handling large volumes of data.
Use Cases
- Data exploration and visualization.
- Predictive modeling and machine learning.
- Text mining and natural language processing.
- Time series analysis and forecasting.
- ETL (Extract, Transform, Load) processes.
User Interface
KNIME provides a user-friendly interface that enables users to build complex data workflows through a visual drag-and-drop approach. Users can connect nodes to define data processing and analysis steps, making it easy to create, modify, and execute workflows.
Pricing
KNIME is an open-source platform and is available for free. However, there are also commercial versions of KNIME, such as KNIME Server and KNIME Server Small, which offer additional features and support. Pricing details for the commercial versions can be obtained directly from the KNIME website.
Pros and Cons
Pros:
- Flexible and customizable platform.
- Large community and extensive library of nodes.
- Integration with external tools and languages.
- Scalability for big data processing.
- Active development and frequent updates.
Cons:
- Steep learning curve for beginners.
- Some advanced features require programming knowledge.
- Limited support for real-time data processing.
- Commercial versions may have additional costs for certain features and support.
Orange
Orange is an open-source data visualization and analysis tool that offers a visual programming interface for data mining and machine learning tasks. It provides a user-friendly environment for data exploration, feature engineering, predictive modeling, and result visualization.
Key Features
- Interactive visual programming interface.
- Wide range of data visualization options.
- Comprehensive collection of data preprocessing and modeling components.
- Integration with machine learning algorithms and libraries.
- Support for data import and export from various formats.
- Interactive data exploration and feature selection.
Use Cases
- Exploratory data analysis.
- Predictive modeling and machine learning.
- Text mining and natural language processing.
- Image analysis and computer vision.
- Time series forecasting.
User Interface
Orange offers an intuitive and interactive visual programming interface. Users can drag and drop components onto a canvas and connect them to create data analysis workflows. The interface allows users to easily manipulate and transform data, build predictive models, and visualize results.
Pricing
Orange is an open-source tool and is freely available for use. There are no costs associated with downloading and using the software. Additionally, Orange offers a range of educational resources and tutorials to support users in utilizing the tool effectively.
Pros and Cons
Pros:
- Intuitive and visually appealing interface.
- Wide range of built-in data preprocessing and modeling components.
- Supports various machine learning algorithms and techniques.
- Extensive library of data visualization options.
- Active community and continuous development.
Cons:
- Limited scalability for handling large datasets.
- Less suitable for big data processing.
- Advanced users may require additional programming knowledge for customization.
- Limited support for some specialized tasks compared to more specialized tools.
Datamelt
Datamelt is an open-source platform for data analysis, scientific computing, and visualization. It provides a comprehensive set of tools and libraries for performing various data-related tasks, including data mining, statistical analysis, machine learning, and numerical computations.
Key Features
- Wide range of mathematical and statistical functions.
- Support for data visualization and plotting.
- Integration with machine learning algorithms and libraries.
- High-performance computing capabilities.
- Extensive collection of data manipulation and transformation tools.
- Connectivity with various data sources and formats.
Use Cases
- Data analysis and exploration.
- Statistical modeling and hypothesis testing.
- Machine learning and predictive analytics.
- Numerical simulations and computational modeling.
- Data visualization and interactive plotting.
User Interface
Datamelt offers a command-line interface (CLI) for executing scripts and commands. It also provides integration with popular programming languages such as Python and Java, allowing users to leverage the capabilities of these languages for data analysis and modeling tasks.
Pricing
Datamelt is an open-source platform and is available for free. There are no costs associated with downloading and using the software. Users can freely access the libraries, tools, and functionalities provided by Datamelt for their data analysis and scientific computing needs.
Pros and Cons
Pros:
- Powerful mathematical and statistical functions.
- Wide range of data visualization options.
- Integration with machine learning algorithms and libraries.
- High-performance computing capabilities.
- Open-source and freely available.
Cons:
- Steep learning curve, particularly for users with limited programming experience.
- Lack of a graphical user interface (GUI) may be less accessible for some users.
- Documentation and community support can be limited compared to more widely used tools.
- Limited pre-built workflows and templates compared to specialized data mining tools.
TIBCO Data Science
TIBCO Data Science is a comprehensive data analytics and machine learning platform that empowers organizations to extract insights, build predictive models, and drive data-driven decision-making. It provides a range of tools and capabilities for data preparation, model development, deployment, and monitoring.
Key Features
- Data exploration and visualization tools.
- Automated machine learning capabilities.
- Integration with popular programming languages and data sources.
- Model development and evaluation functionalities.
- Collaboration and sharing capabilities for team projects.
- Monitoring and management of deployed models.
Use Cases
- Predictive analytics and forecasting.
- Customer segmentation and targeted marketing.
- Anomaly detection and fraud prevention.
- Sentiment analysis and text mining.
- Optimization and decision support.
User Interface
TIBCO Data Science offers a user-friendly visual interface that allows users to create, modify, and execute data analytics workflows. The interface provides drag-and-drop functionality for building data pipelines and model development. Users can also access advanced scripting capabilities for more customized analyses and model development.
pricing
The pricing for TIBCO Data Science varies based on the specific needs and requirements of the organization. TIBCO offers different pricing models, including options for subscription-based licensing. To obtain detailed pricing information, it is recommended to contact TIBCO directly or visit their website.
Pros and Cons
Pros:
- User-friendly interface with drag-and-drop functionality.
- Automated machine learning capabilities for quick model development.
- Integration with external programming languages and data sources.
- Advanced model management and monitoring features.
- Collaboration and sharing functionalities for team projects.
Cons:
- Higher cost compared to some open-source or free alternatives.
- Steep learning curve for beginners.
- Advanced customization may require programming knowledge.
- Some users may find the interface less flexible compared to more code-centric platforms.
- Limited support for big data processing compared to specialized big data analytics tools.
Oracle Data Mining
Oracle Data Mining is a comprehensive data mining solution provided by Oracle Corporation. It is an option within the Oracle Advanced Analytics database feature that enables users to discover patterns, build predictive models, and gain insights from their data.
It integrates seamlessly with Oracle databases, allowing users to leverage the power of data mining within the Oracle ecosystem.
Key Features
- Integration with Oracle databases for seamless data access and processing.
- Advanced data preprocessing and feature engineering capabilities.
- Support for a wide range of machine learning algorithms.
- Scalability for handling large datasets.
- Model evaluation and deployment functionalities.
- Integration with Oracle Business Intelligence tools.
Use Cases
- Customer segmentation and targeted marketing.
- Fraud detection and risk analysis.
- Churn prediction and customer retention.
- Predictive maintenance and asset optimization.
- Market basket analysis and recommendation systems.
User Interface
Oracle Data Mining provides a user interface that is integrated with Oracle SQL Developer, a powerful database development and administration tool. Users can access and execute data mining tasks directly within the SQL Developer environment, making it convenient for database professionals and data analysts.
Pricing
Oracle Data Mining is a commercial offering provided by Oracle Corporation. The pricing structure is based on the specific licensing and usage requirements of the Oracle database and advanced analytics options. For detailed pricing information, it is recommended to contact Oracle directly or consult their official website.
Pros and Cons
Pros:
- Seamless integration with Oracle databases and Oracle SQL Developer.
- Comprehensive set of data preprocessing and modeling tools.
- Extensive library of machine learning algorithms.
- Scalability for handling large datasets.
- Integration with Oracle Business Intelligence tools for advanced analytics and reporting.
Cons:
- Requires Oracle database licensing and infrastructure.
- Steep learning curve for users unfamiliar with Oracle ecosystem and SQL.
- Limited compatibility with non-Oracle databases.
- Some advanced features may require additional licensing or specific configurations.
- Support and documentation may be more focused on Oracle database professionals.
Python
Python is a widely-used, high-level programming language known for its simplicity and readability. It offers a versatile and powerful environment for data mining, analysis, and machine learning tasks. Python has a rich ecosystem of libraries and frameworks that make it a popular choice among data scientists and analysts.
Key Features
- Easy-to-read syntax and code readability.
- Extensive library ecosystem, including NumPy, pandas, scikit-learn, and TensorFlow.
- Support for data manipulation, analysis, and visualization.
- Integration with other programming languages.
- Scalability for handling large datasets.
- Cross-platform compatibility.
Use Cases
- Data preprocessing and cleaning.
- Statistical analysis and hypothesis testing.
- Machine learning and predictive modeling.
- Natural language processing and text mining.
- Data visualization and exploration.
User Interface
Python does not have a specific user interface by default, but it can be executed through various environments, such as Jupyter Notebook, Anaconda, or Integrated Development Environments (IDEs) like PyCharm or Spyder. These environments provide an interactive interface for writing, running, and debugging Python code.
Pricing
Python is an open-source programming language and is available for free. There are no costs associated with using Python itself. However, there may be costs involved when using specific libraries or tools built on top of Python, depending on their licensing or subscription models.
Pros and Cons
Pros:
- Easy-to-learn syntax and code readability.
- Large and active community support.
- Extensive library ecosystem for data analysis, machine learning, and visualization.
- Cross-platform compatibility.
- Integration with other programming languages.
- Suitable for both beginners and experienced developers.
Cons:
- Slower execution speed compared to lower-level languages like C++.
- Requires understanding and managing dependencies between libraries.
- Memory consumption can be high for large-scale data processing.
- Limited support for parallel processing.
- Limited support for GPU acceleration out-of-the-box.
Weka
Weka is a popular open-source data mining and machine learning software written in Java. It provides a comprehensive suite of tools and algorithms for data preprocessing, classification, regression, clustering, association rules, and feature selection. Weka is widely used in both academia and industry for data analysis and predictive modeling tasks.
Key Features
Wide range of data preprocessing and transformation tools.
Support for various machine learning algorithms and techniques.
Integrated tools for data visualization and exploration.
Evaluation and performance analysis of models.
Graphical user interface and command-line interface for flexibility.
Support for integration with other programming languages.
Use Cases
Classification and regression analysis.
Clustering and anomaly detection.
Association rule mining and market basket analysis.
Feature selection and dimensionality reduction.
Text mining and natural language processing.
User Interface
Weka provides a user-friendly graphical user interface (GUI) that allows users to build data mining workflows and interact with the various algorithms and tools. The GUI offers a visual representation of the data flow and allows users to customize and configure the algorithms and parameters.
Pricing
Weka is an open-source software and is freely available for use. There are no costs associated with downloading and using Weka. Users can access the complete set of features and functionalities provided by Weka without any licensing fees.
Pros and Cons
Pros:
- Free and open-source software with a large user community.
- Comprehensive set of data mining and machine learning algorithms.
- User-friendly graphical user interface.
- Extensive data preprocessing and visualization capabilities.
- Integration with other programming languages and tools.
Cons:
- Limited scalability for handling big data compared to specialized big data platforms.
- Steep learning curve for beginners in data mining and machine learning.
- Less suitable for advanced data analytics tasks.
- Limited support for real-time data processing.
- Documentation and user support may vary for some less commonly used algorithms.
Why Data Mining Software Are Essential
Data mining tools are essential for several reasons. First and foremost, these tools enable organizations to extract valuable insights and knowledge from their vast volumes of data. By applying advanced algorithms and statistical techniques, data mining software can uncover hidden patterns, relationships, and trends that may not be apparent through manual analysis.
This allows businesses to make informed decisions, identify opportunities, and mitigate risks based on data-driven insights. Another crucial aspect is the efficiency and scalability that data mining tools offer.
Manual analysis of large datasets can be time-consuming and prone to human error. Data mining tools automate the process, enabling users to process and analyze data at a much faster rate.
These tools also have the capability to handle large volumes of data, making them essential in today's era of big data. With their scalability, businesses can effectively manage and extract insights from complex datasets, leading to improved operational efficiency and informed decision-making.
Moreover, data mining software plays a vital role in various industries and domains. They can be applied in finance for fraud detection and risk assessment, in marketing for customer segmentation and targeted campaigns, in healthcare for disease prediction and diagnosis, and in retail for market analysis and demand forecasting, among many other use cases.
By utilizing data mining tools, organizations can gain a competitive advantage, optimize processes, reduce costs, and drive innovation.
Data Mining Tool Types
There are different types of data mining tools, each serving a specific purpose in the data analysis process. Here are some common types of data mining tools:
- Data Exploration Tools: These tools help users explore and understand the data. They provide functionalities for data profiling, summarization, and visualization, allowing users to gain insights into the characteristics and distribution of the data.
- Data Cleaning Tools: Data cleaning tools focus on data quality and consistency. They assist in detecting and correcting errors, missing values, outliers, and inconsistencies in the data. These tools ensure that the data used for analysis is accurate and reliable.
- Data Preprocessing Tools: Data preprocessing tools transform raw data into a suitable format for analysis. They handle tasks such as data integration, transformation, normalization, and feature selection. These tools prepare the data by addressing issues like data inconsistencies, missing values, and irrelevant features.
- Predictive Analytics Tools: Predictive analytics tools apply statistical and machine learning algorithms to predict future outcomes or behavior based on historical data. These tools enable users to build predictive models for tasks like classification, regression, clustering, and time series forecasting.
- Text Mining Tools: Text mining tools focus on extracting valuable information from textual data. They employ techniques such as natural language processing (NLP) to analyze unstructured text, perform sentiment analysis, entity recognition, topic modeling, and document clustering.
- Visualization Tools: Visualization tools help users visually represent and interpret data. They provide interactive charts, graphs, and dashboards to present complex data in a visually appealing and understandable manner. Visualization tools aid in discovering patterns, trends, and anomalies that may not be evident in raw data.
- Association Rule Mining Tools: Association rule mining tools identify relationships and patterns in large datasets. They discover associations between items and generate rules such as "If A, then B," commonly used in market basket analysis and recommendation systems.
- Clustering Tools: Clustering tools group similar data points together based on their characteristics or attributes. They help identify natural clusters within the data and can be used for segmentation, anomaly detection, and pattern recognition.
Each type of data mining tool serves a unique purpose in the data analysis process, contributing to different stages from data exploration and cleaning to predictive modeling and visualization. By utilizing a combination of these tools, organizations can gain valuable insights and make data-driven decisions.
Conclusion
As we venture into 2023, the landscape of data mining tools and software continues to evolve, offering organizations a multitude of powerful options to extract insights from their data. The best data mining tools for 2023 are characterized by their advanced functionalities, user-friendly interfaces, scalability, and ability to handle large volumes of data.
These tools enable businesses to uncover hidden patterns, relationships, and trends, empowering them to make informed decisions and gain a competitive edge. The selected data mining tools provide a range of capabilities, including data exploration, preprocessing, predictive modeling, visualization, and specialized tasks like text mining and association rule mining.
They cater to various industries and use cases, such as finance, marketing, healthcare, and retail, offering tailored solutions to extract valuable insights from specific domains. With their intuitive interfaces, extensive libraries, and integration with other tools and languages, the best data mining tools for 2023 empower users of all skill levels to effectively analyze and interpret data.
Whether it's for optimizing business processes, improving customer segmentation, detecting fraud, or making data-driven predictions, these tools are indispensable in harnessing the power of data to drive success and innovation in today's data-driven world.
FAQs on Data Mining Tools
What are the upcoming trends in data mining?
Several emerging trends are shaping the field of data mining. Here are some of the upcoming trends:
- Augmented Analytics: Augmented analytics combines machine learning and natural language processing techniques to automate data preparation, analysis, and insights generation. It empowers non-technical users to perform complex data mining tasks with the assistance of AI-driven algorithms, resulting in faster and more accurate insights.
- Explainable AI: As the demand for transparency and accountability in AI algorithms grows, explainable AI is becoming crucial. Explainable AI techniques aim to provide clear explanations and justifications for the decisions made by machine learning models, enhancing trust and enabling better understanding of model predictions.
- Privacy-Preserving Data Mining: With increasing concerns about data privacy, techniques for privacy-preserving data mining are gaining importance. Differential privacy, secure multi-party computation, and federated learning are some approaches that allow data mining to be performed on sensitive data without compromising individual privacy.
These upcoming trends in data mining reflect the evolving needs and challenges of the data-driven era. By embracing these trends, organizations can leverage the power of data mining to drive innovation, make better decisions, and unlock valuable insights from their data.
Which data mining tool is most accessible?
While there are several accessible data mining tools available, Arena Calibrate stands out as one of the most accessible options. Its user-friendly interface and intuitive design make it easy for users, even those without extensive technical expertise, to navigate and utilize its features effectively. Arena Calibrate provides a streamlined experience for data exploration, visualization, and advanced analytics, allowing users to uncover valuable insights from their data with ease.
What are the four main data mining techniques?
The four main data mining techniques are:
- Classification: Classification is a data mining technique used to categorize data into predefined classes or categories based on a set of attributes or features. It involves building a predictive model that assigns new instances to the appropriate class based on their characteristics. Classification algorithms, such as decision trees, support vector machines, and logistic regression, are commonly used for this technique.
- Clustering: Clustering is a technique used to group similar data points together based on their intrinsic characteristics or similarities. It aims to identify natural patterns or clusters in the data without prior knowledge of the class labels. Clustering algorithms, such as k-means, hierarchical clustering, and DBSCAN, are used to partition data into meaningful clusters.
- Association Rule Mining: Association rule mining discovers relationships or associations between items in large datasets. It focuses on finding interesting patterns, often referred to as association rules, which describe the co-occurrence or dependency between items. This technique is commonly used in market basket analysis and recommendation systems. Apriori and FP-growth are popular algorithms for association rule mining.
- Regression: Regression is a data mining technique used to model the relationship between a dependent variable and one or more independent variables. It aims to predict continuous numeric values based on the input variables. Regression algorithms, such as linear regression, polynomial regression, and support vector regression, are employed to build models that estimate the value of the dependent variable.
How to select the best data mining tool?
Selecting the best data mining tool involves considering several factors based on your specific needs and requirements. Here are some key steps to help you in the selection process:
- Define your objectives: Clearly outline your goals and objectives for data mining. Identify the specific tasks you need to perform, such as classification, clustering, predictive modeling, or text mining. Understanding your objectives will help you evaluate whether a tool aligns with your needs.
- Assess data requirements: Consider the characteristics of your data, such as volume, complexity, and format. Determine if the tool can handle your data size and type. Some tools specialize in handling big data or unstructured data, while others are more suitable for smaller datasets or structured data.
- Evaluate functionality and features: Review the functionalities and features offered by the data mining tool. Look for capabilities such as data preprocessing, visualization, machine learning algorithms, model evaluation, and integration options with other tools or programming languages. Ensure that the tool provides the necessary functionalities for your specific data mining tasks.
- Consider ease of use and learning curve: Evaluate the user-friendliness of the tool's interface. Consider your own technical expertise and the skill level of the intended users. Look for tools with intuitive interfaces, drag-and-drop functionality, and comprehensive documentation or tutorials to facilitate learning and usage.
- Community and support: Consider the availability of a supportive user community, forums, and resources associated with the data mining tool. A vibrant community can provide assistance, guidance, and shared experiences, which can be valuable when using the tool or encountering challenges.